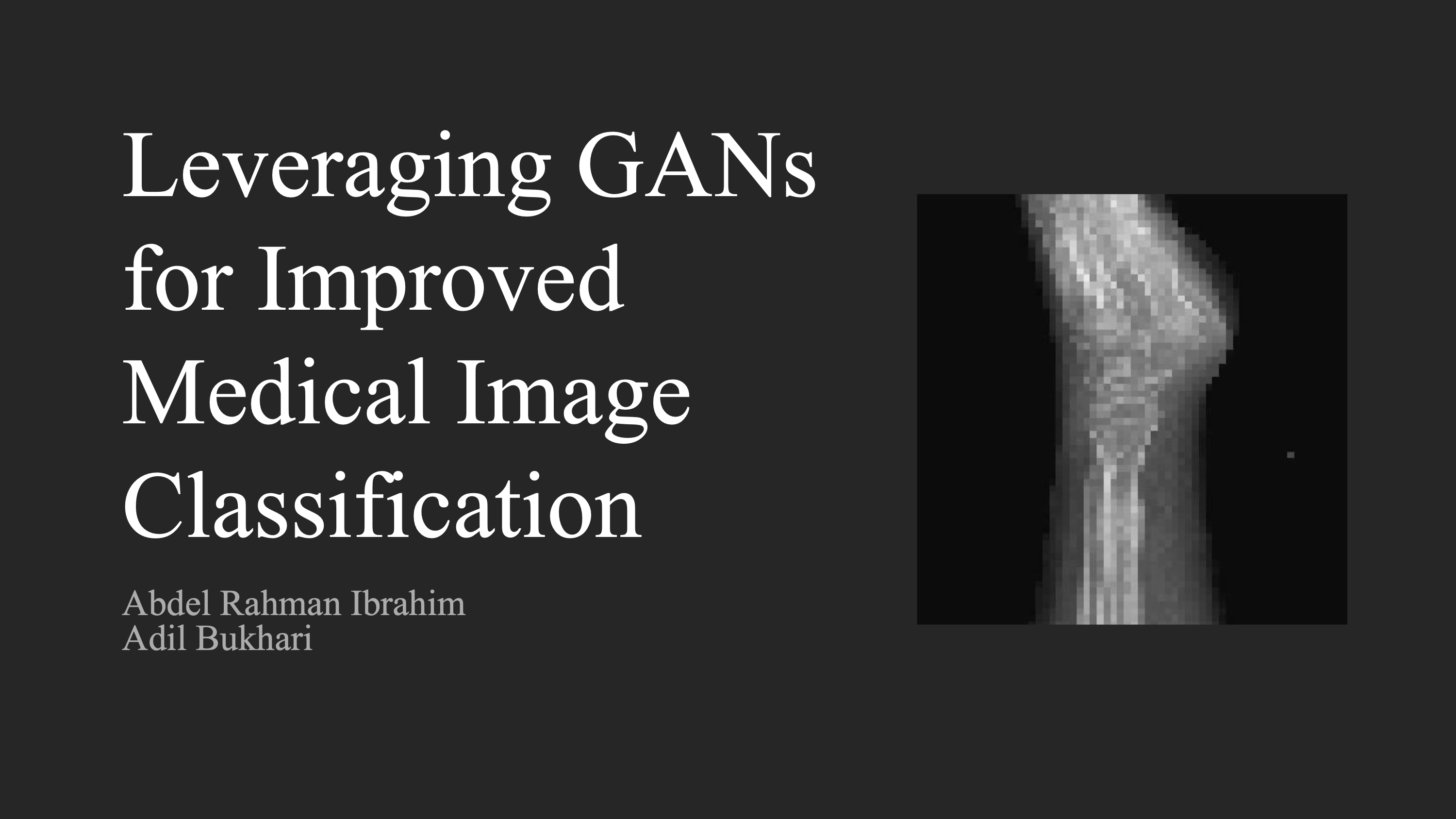
Leveraging GANs for Improved Medical Image Classification
Using GANs to generate synthetic data for augmenting training datasets, improving model robustness, and addressing challenges in collecting large-scale, labeled data, especially in constrained domains like medical imaging.
Problem & Motivation
In numerous machine learning applications, the availability of labeled training data plays a crucial role in model performance. However, collecting large-scale, labeled datasets that accurately represent the underlying data distribution can be expensive and time-consuming. Furthermore, in domains such as medical imaging where data acquisition is constrained by ethical and privacy concerns, collecting high-quality data is even more difficult.
Proposal
We propose leveraging Generative Adversarial Networks (GANs) for synthetic data generation to address the aforementioned challenges. By training GANs on existing labeled data, we can generate synthetic data samples that closely resemble the true data distribution. These synthetic samples can then be used to augment the original dataset, effectively increasing the diversity and size of the training dataset. Additionally, GAN-generated data can help prevent overfitting by introducing variability into the training process and improving model robustness against unseen data samples.
Datasets
We will be utilizing the Bone Fracture Multi-Region X-Ray Dataset found here. This dataset contains ~10,000 x-ray images of fractured and non-fractured bones covering all anatomical body regions.
Methods
Using a labeled dataset of real images, we will train a GAN to generate synthetic data that closely resembles the real images from the dataset. The usability of this data for model training will be evaluated by training an existing image classification model with and without the inclusion of synthetic data and using a subset of the original dataset as testing data to compare the models’ performance on classification tasks.
We will be training two GAN models, each tailored for generated positive/negative samples of fractured image data. Using these, we will generate ~10,500 synthetic samples of fractured and unfractured images. These samples will be used alongside the original training dataset in our experimental process for training the bone fractures image classifier.
Model Architecture
The architecture of the CNN model used in the experiment is as follows:
┏━━━━━━━━━━━━━━━━━━━━━┳━━━━━━━━━━━━━┳━━━━━━━━━━┓
┃ Layer (type) ┃ Output Shape┃ Param ## ┃
┡━━━━━━━━━━━━━━━━━━━━━╇━━━━━━━━━━━━━╇━━━━━━━━━━┩
│ Conv2D │ (21, 21, 32)│ 320 │
│ BatchNorm │ (21, 21, 32)│ 128 │
│ Conv2D │ (7, 7, 16) │ 4,624 │
│ MaxPooling2D │ (2, 2, 16) │ 0 │
│ BatchNorm │ (2, 2, 16) │ 64 │
│ Dropout │ (2, 2, 16) │ 0 │
│ Flatten │ (64) │ 0 │
│ Dense │ (128) │ 8,320 │
│ Dropout │ (128) │ 0 │
│ Dense │ (1) │ 129 │
└─────────────────────┴─────────────┴──────────┘
Total params: 13,585
The architecture of the generator and discriminator components is as follows:
Generator:
┏━━━━━━━━━━━━━━━━━━━━━┳━━━━━━━━━━━━━━━┳━━━━━━━━━━━┓
┃ Layer (type) ┃ Output Shape ┃ Param ## ┃
┡━━━━━━━━━━━━━━━━━━━━━╇━━━━━━━━━━━━━━━╇━━━━━━━━━━━┩
│ Dense │ (8192) │ 1,056,768 │
│ Reshape │ (8, 8, 128) │ 0 │
│ Conv2DTranspose │ (16, 16, 128)│ 262,272 │
│ LeakyReLU │ (16, 16, 128)│ 0 │
│ Conv2DTranspose │ (32, 32, 256)│ 524,544 │
│ LeakyReLU │ (32, 32, 256)│ 0 │
│ Conv2DTranspose │ (64, 64, 512)│ 2,097,664 │
│ LeakyReLU │ (64, 64, 512)│ 0 │
│ Conv2D │ (64, 64, 3) │ 38,403 │
└─────────────────────┴──────────────┴────────────┘
Total params: 3,979
Discriminator:
┏━━━━━━━━━━━━━━━━━━━━━┳━━━━━━━━━━━━━━┳━━━━━━━━━━┓
┃ Layer (type) ┃ Output Shape ┃ Param ## ┃
┡━━━━━━━━━━━━━━━━━━━━━╇━━━━━━━━━━━━━━╇━━━━━━━━━━┩
│ Conv2D │ (32, 32, 64) │ 3,136 │
│ LeakyReLU │ (32, 32, 64) │ 0 │
│ Conv2D │ (16, 16, 128)│ 131,200│
│ LeakyReLU │ (16, 16, 128)│ 0 │
│ Conv2D │ (8, 8, 128) │ 262,272 │
│ LeakyReLU │ (8, 8, 128) │ 0 │
│ Flatten │ (8192) │ 0 │
│ Dropout │ (8192) │ 0 │
│ Dense │ (1) │ 8,193 │
└─────────────────────┴──────────────┴──────────┘
Total params: 404,801
Experiments
As part of our investigation, we conduct four distinct experiments to examine the impact of incorporating synthetic data during training on the generalizability and performance of the classifier model.
Experiment 1: Train CNN with real training dataset
Experiment 2: Train CNN with augmented training dataset
Experiment 3: Train CNN with combined real and synthetic datasets
Experiment 4: Train CNN on real dataset after warm starting it with synthetic data
The experimental setup can be found here: ECS289L_CNN_Experiments.ipynb
Results
For each of the experiments, we trained a basic CNN on the respective datasets for 20 epochs. The results were as follows:
- Experiment 1: Accuracy of 92%
- Experiment 2: Accuracy of 87%
- Experiment 3: Accuracy of 91%
- Experiment 4: Accuracy of 93%
The accuracy and loss curves for the experiments are depicted in the charts below.
Experiment 1:
Experiment 2:
Experiment 3:
Experiment 4:
Discussion
The experiments show that training with the original dataset on a model that was warm-started with synthetic data (Experiment 4) yielded the highest accuracy. The results of experiments 2–4 were benchmarked against training the classifier on the original dataset.
It was interesting to see that training with an augmented dataset using simple transforms (Experiment 2) actually hindered the model’s performance. It seemed that the more transforms we applied (zoom, flips, etc.), the worse the accuracy got. We concluded that this is likely due to the very controlled conditions that medical images such as X-Rays are taken in. Any divergence from this seems to inhibit the model’s ability to classify the image data.
Experiment 3, in which we trained on a combined dataset containing the synthetic and real images, performed almost as well as Experiment 1. It’s probable that this was due to the GANs not producing as high quality synthetic images as we would have liked.
Experiment 4 was the only one to perform better than training on the original dataset. The model was likely able to pick up features from the synthetic data and avoided needing to start from scratch when being trained on the real image data which would explain the increase in accuracy.
Future Work
For future research, we propose using more recent and advanced GAN architectures (Ex: StyleGAN, CycleGAN) to generate higher quality synthetic images. It would be interesting to see if this, perhaps in combination with the warm-starting technique used in Experiment 4, could lead to more significant increases in model performance.