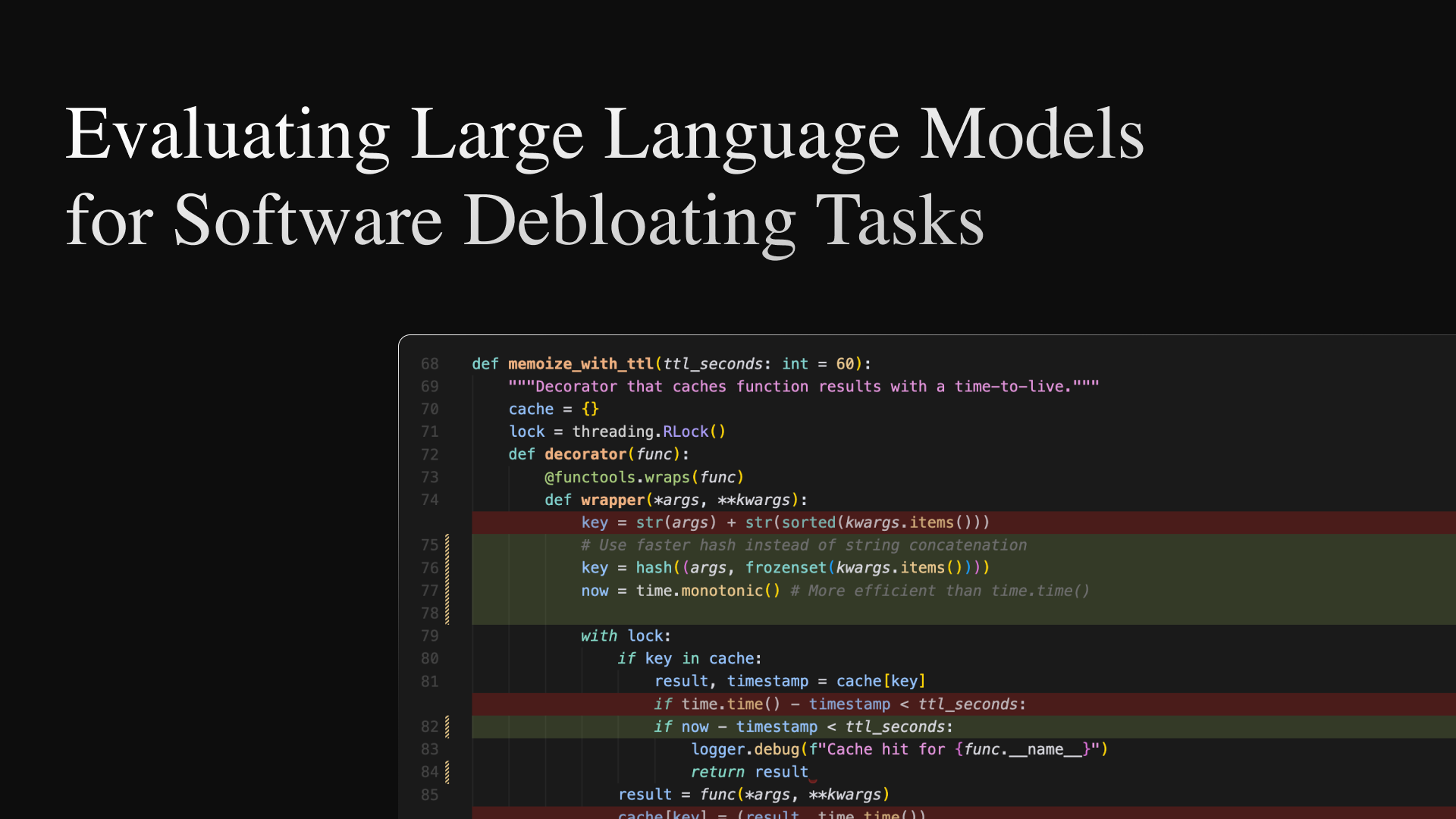
Evaluating Large Language Models for Software Debloating Tasks
Abstract
Software bloat—excessive or inefficient code that increases program size and reduces performance—continues to provide a considerable challenge in contemporary software development. This study investigates the efficacy of Large Language Models (LLMs) in identifying and eliminating software bloat without a loss functionality. We evaluated the performance of various LLMs including GPT-4, Claude 3.5 Sonnet, and DeepSeek v3, on 76 Python code files from 26 repositories through empirical evaluations. According to our data, while LLMs can considerably reduce code size (GPT-4o achieved the highest average reduction of 25 64%), this often comes at the cost of functional accuracy and execution time. Claude 3.5 sonnet was the most reliable of the models we tested, passing 97.11% of test cases, although with more conservative reductions. Further prompt engineering experiments demonstrate the potential improvement of the observed optimization results. These results provide insights in finding the appropriate balance between software dilution and the software itself, effectively defining meaningful roles for LLMs in the software design and development process.
Read the full paper here: [PDF Link]